The Race for AI eXperience
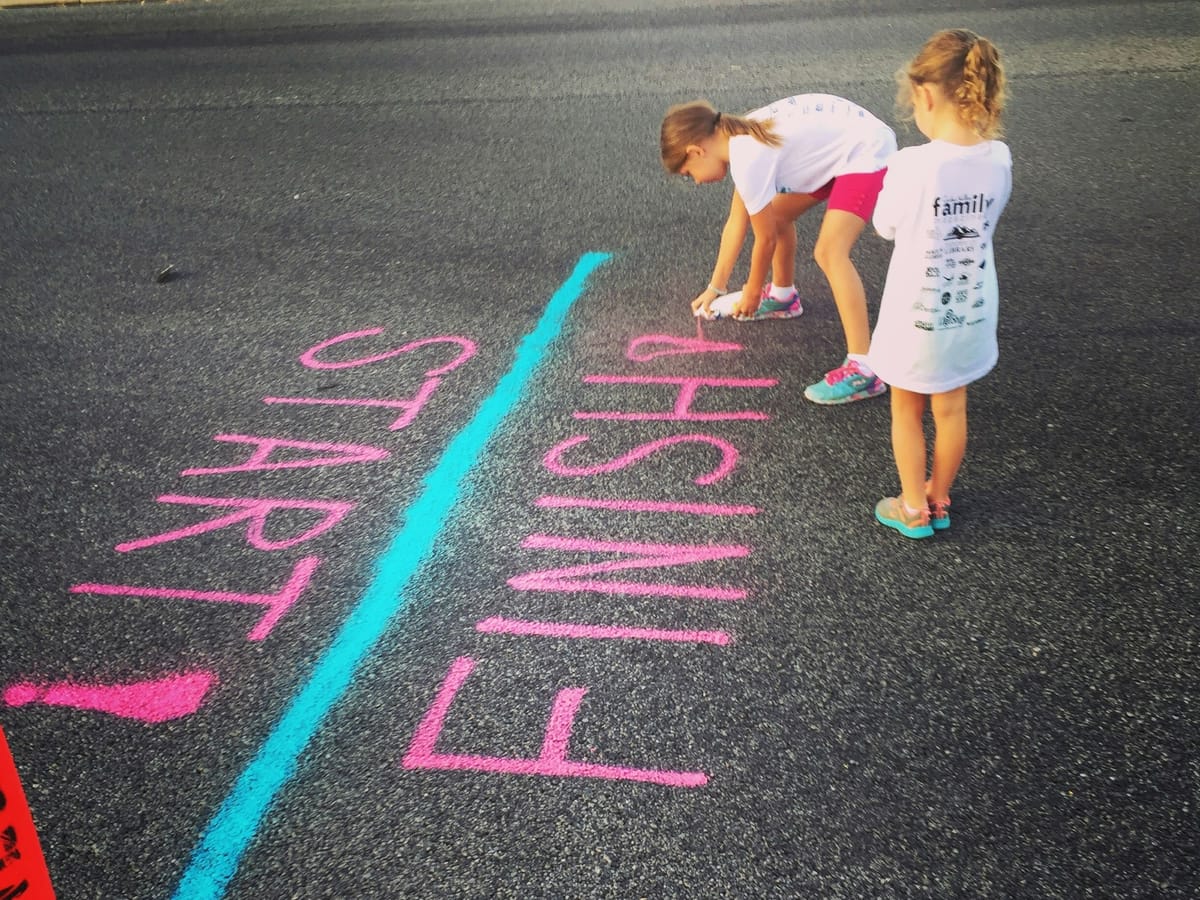
"Crypto AI agents have taken the starting pistol and put it to their head"
In the rapidly evolving world of crypto, a new breed of AI-powered digital assistants has emerged, promising to revolutionise how we interact with DeFi. Yet despite the hype and flashy marketing campaigns touting "AI agents", the reality falls dramatically short of expectations. The race for true AI eXperience (AIX) has begun, but most have stumbled before crossing the starting line.
The AIX Paradox
Current crypto AI agents face a fundamental paradox: they must choose between being fast but ineffective, stable but limited or powerful but prohibitively slow and expensive. This problem has led to agents failing to deliver meaningful value beyond social agents.
When users interact with digital assistants, they expect to feel connected with immediate responses. However, all language models, from GPT-4o to Claude 3.7 to smaller variants like GPT-4o mini, suffer from significant "Time To First Token" (TTFT) delays. Each API call introduces at least 4 seconds of delay before generating even a single token of response. This latency compounds dramatically during multi-turn operations involving tool use, searches, or chain-of-thought reasoning.
The problem is worse with tool use, as every tool costs +4 seconds. For chain operations requiring multiple sequential steps: checking prices, analysing trends, executing trades - these delays cascade into an experience that feels sluggish and disconnected.
Technical Barriers Creating Friction
Beyond the speed / capability tradeoff, several technical limitations hamper today's crypto agents. The standard approach to tool usage, where agents interact with external data sources and blockchains, relies on JSON formatting that is notoriously error-prone. A single misplaced comma can break functionality entirely.
The context window problem further complicates matters. While models advertise impressive context lengths (Claude boasts 200K tokens), yet performance degrades dramatically after about 50K tokens. When agents pull in blockchain data, market information, and other necessary trifles, they quickly exhaust this effective context window, leading to degraded performance.
Multi-agent architectures are another attempted solution that compounds these problems. Each agent in the chain adds its own TTFT, creating a cumulative delay that makes complex operations painfully slow for users.
The Economics Don't Add Up
Building truly effective agents using premium models like GPT-4 ($8 per million tokens) makes scaling economically unfeasible. Lower-cost alternatives lack the stability and reasoning capabilities needed for financial operations where mistakes can be costly.
Self-hosting open-source models might seem like a solution, but this approach becomes unmanageable at scale, particularly for startups without large data centres.
The result? Current market offerings and frameworks have effectively "committed suicide at the starting line" by choosing model combinations that cannot possibly deliver on their promises.
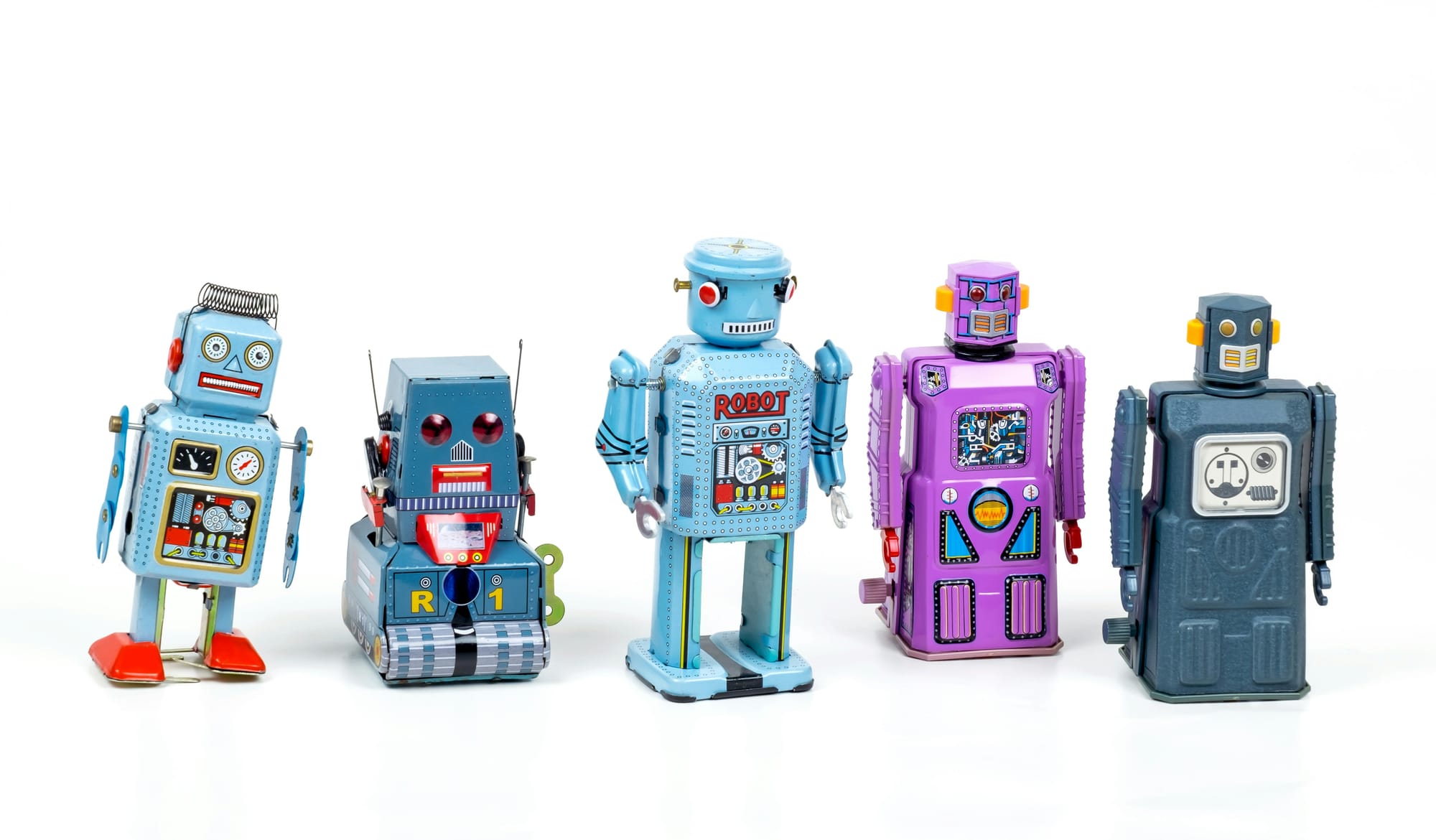
A New Approach: Data + Framework
The path forward requires rethinking how AI agents are constructed from the ground up. Instead of chasing ever-larger context windows or more expensive models, the solution lies in combining high-performance data delivery with purpose-built frameworks.
What does this look like in practice?
First, strategic model selection is crucial. Models from 8B to 70B parameter range (like those offered by Groq) hit the sweet spot between capability and cost, particularly when they offer close to zero TTFT.
Second, purpose-built frameworks must replace academic solutions that don't translate to production environments. Avoiding pitfalls with poorly formatted JSON, manage context efficiently, and incorporate specialised reasoning modules that provide stability without excessive "think time."
Third, data delivery must become a first-class concern. Getting the right information to the model at the right time, in the right format, dramatically improves performance without requiring slow reasoning models or large context windows.
By combining fast data delivery with purpose-built frameworks and strategically selected models is the only way we will experience truely useable digital assistants. Ones that are stable, fast, and cost-effective, and work for you whilst you're asleep.
As our industry evolves, the winners in this race won't be determined by marketing budgets or token launches but by creating AIXs that work.
The starting gun has fired, and while many contenders have already faltered, the race has just begun.